Moving Beyond AI Bias: How the Right Talent Solves the Hard Problems
Bias in AI isn’t a new problem.
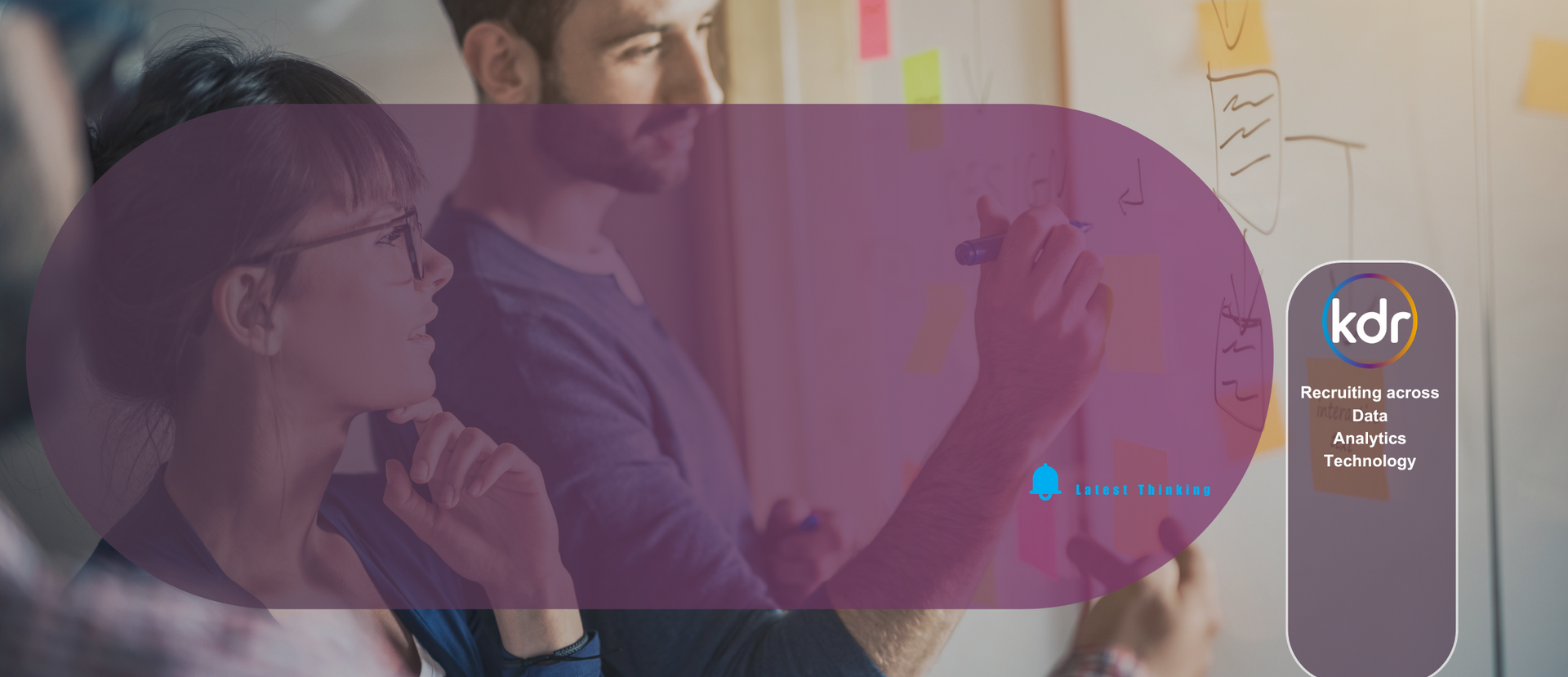
If you’re working in machine learning, computer vision or data science, you’ve likely already encountered the challenges around data imbalance, opaque models or underperforming systems across different demographic groups.
What matters now is what we do about it and more importantly, who we hire to fix it going forward. Most AI bias issues don’t stem from bad intentions, they come from gaps in team capability or oversight. So, what is the solution? Building multidisciplinary teams that are proactive, not reactive. That means hiring not just engineers and data scientists, but ethics-trained ML practitioners who can apply fairness metrics and bias mitigation techniques during development, not after the fact. On top of this, data curation experts who understand representation, annotation quality and provenance and then product and policy professionals who can balance innovation with governance and regulatory frameworks.
This isn’t about hiring generalists who “care about bias.” It’s about embedding specialist roles at every stage of the model lifecycle.
Standards are catching up, so should your talent strategy. With the launch of ISO/IEC 42001:2023, AI governance has a new global benchmark and this standard helps organisations build structured AI management systems with clear responsibilities, continuous monitoring, and ethical oversight.
But as we all know, a framework is only as good as the people implementing it. In my opinion, hiring individuals who understand (or can upskill into) ISO-aligned practices will be critical. Whether that’s a responsible AI lead who maps ethical risks, or an MLOps engineer integrating fairness evaluations into pipelines, the ability to align people with processes is where real progress will be made.
The most impressive AI teams I speak to right now aren’t waiting for regulation to force their hand. They’re running a variety of checks:
- Running bias audits as a core KPI, not as an afterthought.
- Pairing research scientists with ethicists in model validation.
- Incentivising teams to publish fairness benchmarks alongside performance metrics.
- Prioritising candidates with experience in causal inference, adversarial testing, or explainable AI, not just Kaggle scores.
And I believe, this mindset shift is changing the game from compliance to competitive advantage.
Where we come in
At KDR, we’re not just helping organisations find AI talent, we’re helping them find the right talent to build trustworthy, future-proof systems. I work closely with candidates who are actively shaping the ethical AI space, and who want to work for companies taking these issues seriously.
If you’re looking to scale a team that’s technically sharp and ethically aware, or if you’re struggling to find that niche mix of applied ML and governance expertise, we can help.
Because fixing AI bias isn’t just a technical problem. It’s a talent problem. And solving it starts with who you hire.